Shifts in technology such as AI and deep learning have emerged as revolutionary tools for transformation. Traditional ways of using deep learning in the AI industry were limited, but now it is being utilized in more creative fields such as fashion and even sensitive AI Nudify offerings. This shift in how technology is approached enhances user experience, while also raising important challenges related to ethics, privacy and consent. Through deep learning, an advanced set of algorithms, vast sets of data are analyzed allowing for many industry sectors to reproduce human-like reactions. It has become evident that these types of systems are starting to change the face of technological industries and impact human-machine relations. This article will discuss the use of deep learning in AI, its applications and ethical implications.
Understanding Deep Learning
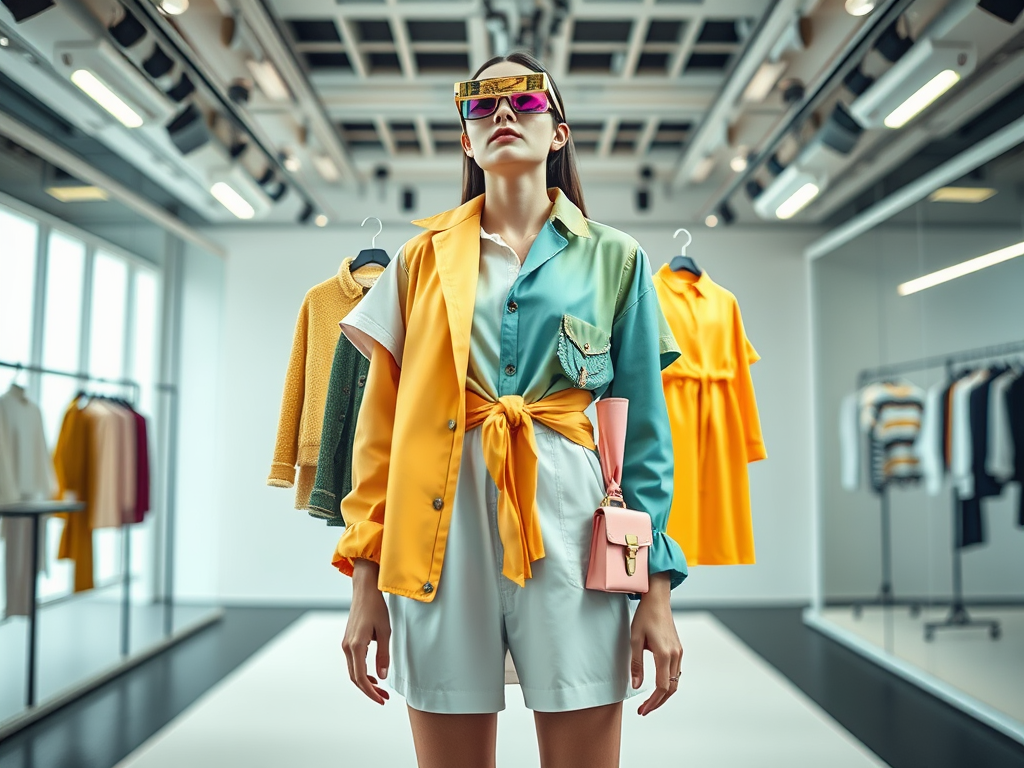
What is Deep Learning?
Deep learning is a sub-field of machine learning that employs neural networks for data analysis. Deep learning is different from conventional machine learning algorithms because it can process large volumes of unstructured data. In the same way that the human brain works, deep learning uses neural networks which are layered structures to try an understand and models’ processes. What sets deep learning apart from other conventional methods is its automatic feature extraction ability. The model does not need intensive feature engineering since through the big data it is exposed to builds the model on its own. This advancement increases the level of applications in several fields and boosts speed and precision.
The Architecture of Deep Learning
The construction of a neural network in deep learning consists of an intricate arrangement of multiple neuron layers that receive and process data. Each architecture is characterized by its depth (number of layers) and breadth (number of neurons per layer). The most widely used models in deep learning include hybrid forms of Convolutional Neural Networks (CNN) and Recurrent Neural Networks (RNN), as well as their individual variants. While RNNs are sequence prediction oriented, CNNs are good at image processing which makes them suitable for applications such as virtual try-on systems. The architecture type selected will inform the impact and performance metrics of a model. With the proper configurations of the neurons and layers, the desired deep learning system can be achieved by developers.
Applications of Deep Learning in AI
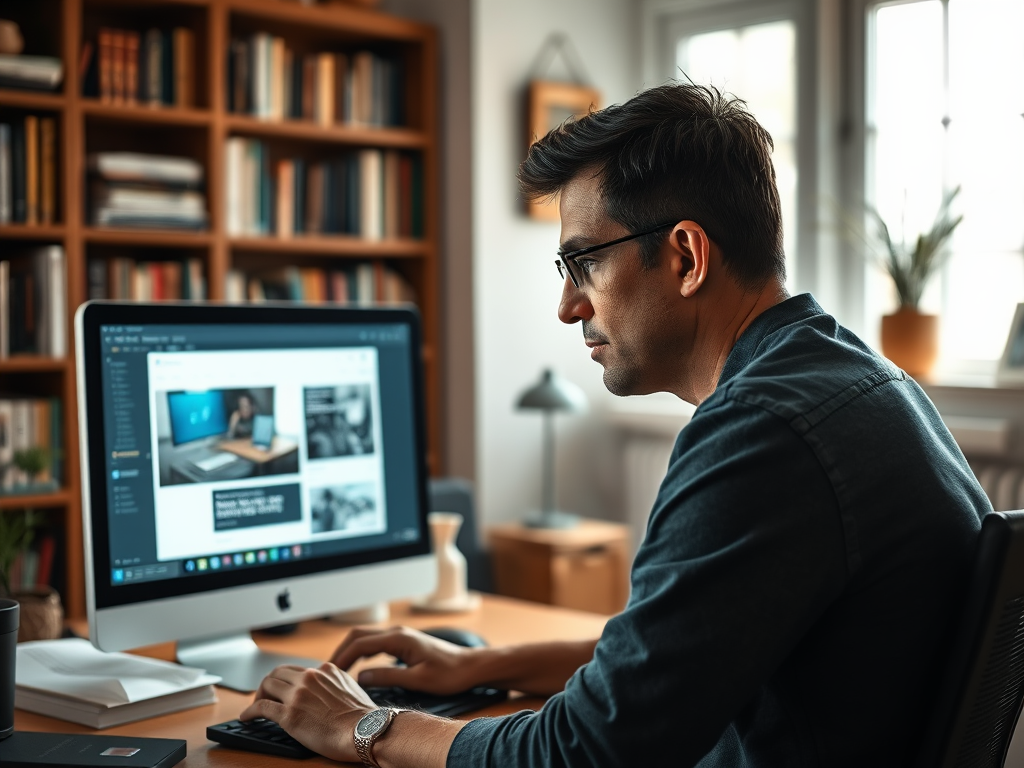
Virtual Try-Ons: Revolutionizing the Fashion Industry
Deep learning has been put to some of the most interesting use cases, such as virtual try-ons where consumers can see how certain clothes would look on them, eliminating the need for them to actually put on the clothes. This means fewer returns and an overall better experience for consumers. In terms of implementation, a lot of online merchants are putting AI and AR in their virtual storefronts which makes the shopping experience more engaging.
With these new technologies, consumer confidence is on the rise, which is helping businesses expand and establish themselves in an ever more competitive environment.
Mechanics Behind Virtual Try-Ons
The processes associated with virtual try-ons are based on the implementation of convolutional neural networks (CNNs) in addition to other machine learning models. These systems first parse user images and then perform texture mapping so that the garment may be rendered on the person. Most applications proceed as follows:
- Image capture of the user’s body
- Facial recognition for proper alignment
- Clothing texture analysis
- Simulation of clothing movement and fit based on user posture
This multi-step process results in highly realistic representations, significantly improving user experiences. As this technology evolves, retailers can expect to see increased sales and reduced return rates.
Technology | Application | Target Industry |
---|---|---|
Convolutional Neural Networks | Virtual Try-Ons | Fashion E-commerce |
Recurrent Neural Networks | Natural Language Processing | Customer Service |
Generative Adversarial Networks | Nudify AI | Content Creation |
Nudify AI: Controversies and Ethical Implications
However, deep-learning technologies have also been integrated into more controversial tools like Nudify AI, which is an ethical nightmare in many aspects, including privacy as well as consent. Nudify AI utilizes neural network algorithms which are capable of performing explicit transformations of images without the consent or knowledge of the subject. Emerging from this technology are concerns of possible abuse and exploitation. These technologies carry such great risk that the establishment of ethical boundaries is imperative to ensure no harm is inflicted. Organizations need to prioritize consent and ensure ethical compliance throughout the development and application of AI technologies.
The Need for Regulation
The advent of technologies such as Nudify AI has underscored the urgent need for comprehensive regulations governing AI practices. As technologies evolve, so too must the frameworks that oversee their use. Essential steps in this process include:
- Implementation of strict privacy laws
- Creation of ethical standards for AI developers
- Public awareness campaigns regarding the risks of AI technologies
By focusing on regulation, society can ensure the responsible use of powerful technologies while advancing innovation in a safe manner.
The Future of Deep Learning in AI
In the coming years, the prospects in deep learning seem boundless. There is constant work being done to improve algorithms, increasing their learning speed and precision. Incorporation of deep learning in augmented and virtual reality is very promising. Moreover, with AI adoption on the rise, competition for skilled professionals in the tech industry will most probably intensify. The impact on healthcare, automotive, education, and others is profound. As innovation continues within industries, new use cases around the advancements made through deep learning could arise.
Conclusion
To conclude, deep learning is emerging as one of the most advanced facets in AI, tailormaking our confrontation with technology in various sectors. This technology also depicts a double-edged sword from transforming the online shopping experience with virtual try-ons to ethical dilemmas such as Nudify AI. Integrating conversations of deep learning usage need to encase issues of responsibility and potential repercussions. There are reasons to believe coming advances will irrevocably change the relations within society and AI-technology, thus underlining the need for ethical scrutiny when tackling this enthusiasm-loaded region.
Frequently Asked Questions
- What is the difference between traditional AI and deep learning? Traditional AI often employs rule-based algorithms, whereas deep learning mimics human brain processes through neural networks to analyze complex data patterns.
- How do virtual try-ons work? Virtual try-ons utilize facial recognition and body tracking technologies powered by deep learning algorithms to create realistic representations of clothing on users.
- What are the ethical concerns surrounding Nudify AI? The ethical concerns include privacy violations, consent issues, and the potential for misuse in the creation of non-consensual explicit images.
- Can deep learning be used in other industries? Yes, deep learning has applications in various fields, including healthcare, finance, and autonomous vehicles, among others.
- What advancements can we expect in deep learning in the coming years? Future advancements may involve improved training techniques, better handling of biases in AI models, and increased integration of explainability in deep learning systems.